Table Of Content
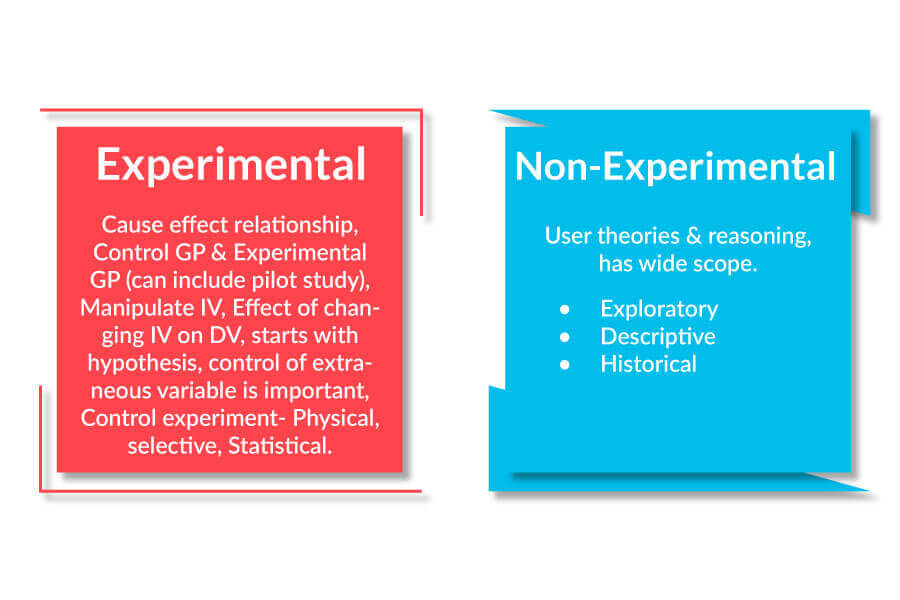
Instead of the attractive condition always being first and the unattractive condition always being second, the attractive condition comes first for some participants and second for others. Likewise, the unattractive condition comes first for some participants and second for others. Thus any overall difference in the dependent variable between the two conditions cannot have been caused by the order of conditions. A second way to think about what counterbalancing accomplishes is that if there are carryover effects, it makes it possible to detect them.
Step 3: Design your experimental treatments
Furthermore, you need to clearly state how your research will contribute to the research field, either by adding value to the pertinent literature or challenging previous findings and assumptions. When groups experience different product designs, the company can assess which option most appeals to potential customers. When you have a clear idea of how to carry out your experiment, you can determine how to assemble test groups for an accurate study. Determine which type of stimulus meets your experiment’s needs and how widely or finely to vary your stimuli.
What is experimental research design?
In a within-subjects experiment, however, the same group of participants would judge the guilt of both an attractive and an unattractive defendant. Experimental design also allows researchers to generalize their findings to the larger population from which the sample was drawn. By randomly selecting participants and using statistical techniques to analyze the data, researchers can make inferences about the larger population with a high degree of confidence. This design involves grouping participants within larger units, such as schools or households, and then randomly assigning these units to different treatment groups. The two variable groups mirror the control groups, but researchers expose them to stimuli.
Pre-Experimental Design Cons
Sometimes, recruiting a sample of people to randomly assign may be difficult. The controlled nature means the research findings may not fully apply to real-world situations or people outside the experimental setting. Experimental design is a research method that enables researchers to assess the effect of multiple factors on an outcome. Let's say researchers are testing a new medication for high blood pressure.
While Field Experiments offer real-world relevance, they come with challenges like controlling for outside factors and the ethical considerations of intervening in people's lives without their knowledge. Field Experiments embrace the messiness of the real world, unlike laboratory experiments, where everything is controlled down to the smallest detail. Last but certainly not least, let's explore Field Experiments—the adventurers of the experimental design world.
Simultaneous Within-Subjects Designs
Of all the types, the simplest type of experimental design is the completely randomized design, in which the participants are randomly assigned to the treatment groups. The main advantage of using this method is that it avoids bias and controls the role of chance. This method provides a solid foundation for Statistical analysis as it allows the use of probability theory.
A Quick Guide to Design Rigorous Machine Learning Experiments - Towards Data Science
A Quick Guide to Design Rigorous Machine Learning Experiments.
Posted: Wed, 08 Feb 2023 08:00:00 GMT [source]
Imagine wanting to know how people of different ages feel about a new video game. Instead of waiting for years to see how opinions change, you could just ask people of all ages what they think right now. Plus, keeping track of participants over many years can be like herding cats—difficult and full of surprises. So next time you read about a groundbreaking discovery in medicine or technology, chances are a True Experimental Design was the VIP behind the scenes, making sure everything was on point. It's been the go-to for rigorous scientific inquiry for nearly a century, and it's not stepping off the stage anytime soon.
The same is true for intervening variables (a variable in between the supposed cause (X) and the effect (Y)), and anteceding variables (a variable prior to the supposed cause (X) that is the true cause). When a third variable is involved and has not been controlled for, the relation is said to be a zero order relationship. In most practical applications of experimental research designs there are several causes (X1, X2, X3). In a quasi-experimental design, the participants of the groups are not randomly assigned.
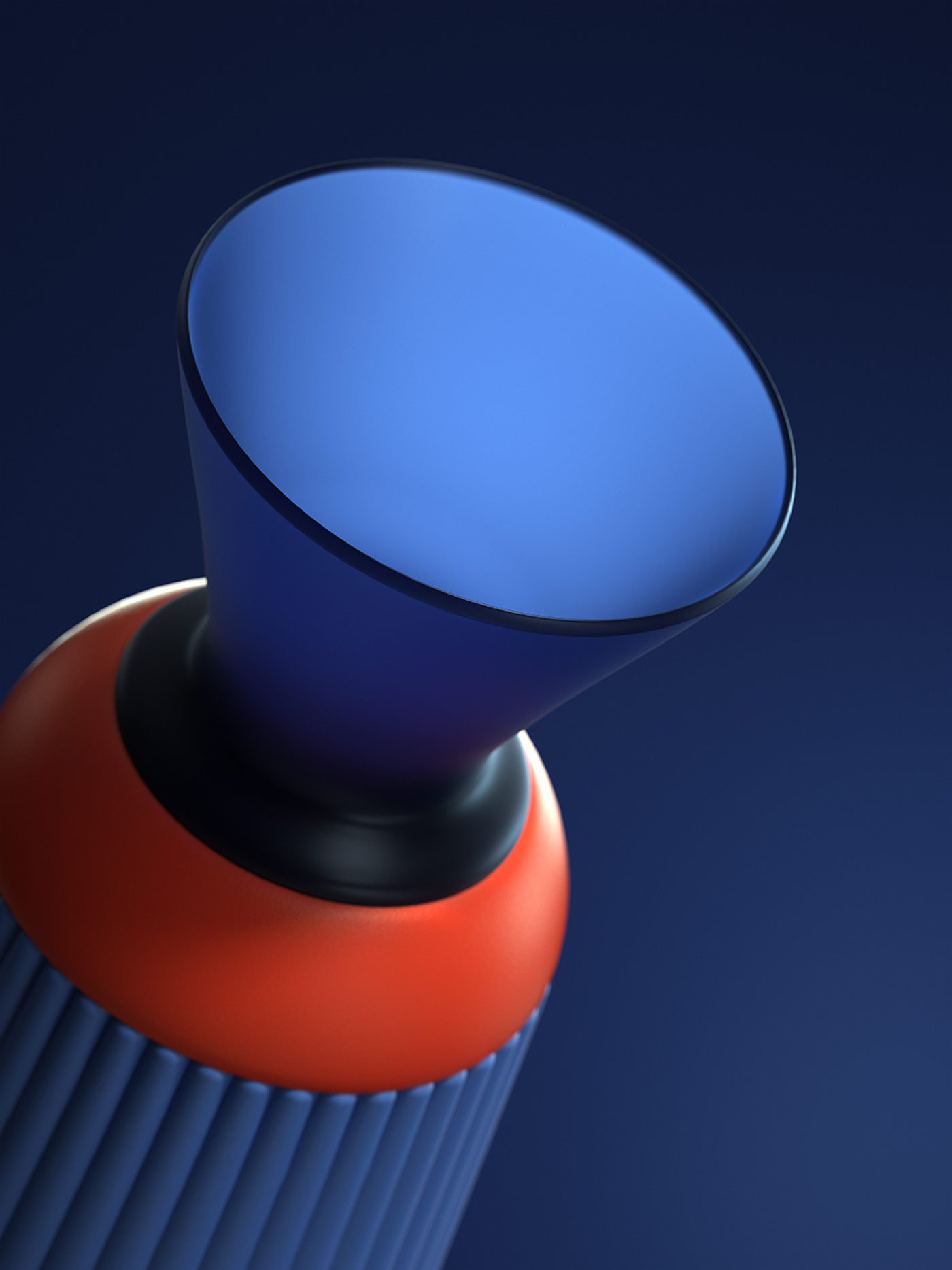
It may involve the use of structured or unstructured observation, and may be conducted in a laboratory or naturalistic setting. Replication involves conducting the same experiment with different samples or under different conditions to increase the reliability and validity of the results. Blinding involves keeping participants, researchers, or both unaware of which treatment group participants are in, in order to reduce the risk of bias in the results. I learned a lot about the Strategy of experimentation and basic statistical concepts. While experimental research might be the right choice for some studies, certain conditions could render experiments useless or even dangerous.
In addition to classic experimental design, there are two other ways of designing experiments that are considered to fall within the purview of “true” experiments (Babbie, 2010; Campbell & Stanley, 1963). The posttest-only control group design is almost the same as classic experimental design, except it does not use a pretest. Researchers who use posttest-only designs want to eliminate testing effects, in which participants’ scores on a measure change because they have already been exposed to it. If you took multiple SAT or ACT practice exams before you took the real one you sent to colleges, you’ve taken advantage of testing effects to get a better score. Considering the previous example on racism and depression, participants who are given a pretest about depression before being exposed to the stimulus would likely assume that the intervention is designed to address depression. That knowledge could cause them to answer differently on the post-test than they otherwise would.
Picture a scientist leaving the controlled environment of a lab to test a theory in the real world, like a biologist studying animals in their natural habitat or a social scientist observing people in a real community. These are Field Experiments, and they're all about getting out there and gathering data in real-world settings. Think of Sequential Design as the nimble athlete of experimental designs, capable of quick pivots and adjustments to reach the finish line in the most effective way possible. But just like an athlete needs a good coach, this design requires expert oversight to make sure it stays on the right track. In practical terms, this design is often seen in clinical trials for new drugs or therapies, but its principles are also applicable in fields like psychology, education, and social sciences.
We discuss what 'covariates' are, how they help, and the kinds of biological insight they can provide when their measurement is included in an experimental design and analysis. That said, our current discussion of experimental design only tangentially addresses some fundamental topics. For example, we provide thorough discussion of power analysis for a variety of experimental designs (see later Chapters) to help researchers decide upon the appropriate level of replication (sample size) for their experiment.
This experimental design method involves manipulating multiple independent variables simultaneously to investigate their combined effects on the dependent variable. Sometimes randomisation isn’t practical or ethical, so researchers create partially-random or even non-random designs. An experimental design where treatments aren’t randomly assigned is called a quasi-experimental design. (Does the attractiveness of one person depend on the attractiveness of other people that we have seen recently?) But when they are not the focus of the research, carryover effects can be problematic.
Researchers now use super powerful software to help design their experiments and crunch the numbers. Around 350 BCE, people like Aristotle were trying to figure out how the world works, but they mostly just thought really hard about things. So while they were super smart, their methods weren't always the best for finding out the truth. A confounding variable could be an extraneous variable that has not been controlled. Repeated Measures design is also known as within-groups or within-subjects design. This should be done by random allocation, ensuring that each participant has an equal chance of being assigned to one group.
Imagine, for example, that participants judge the guilt of an attractive defendant and then judge the guilt of an unattractive defendant. If they judge the unattractive defendant more harshly, this might be because of his unattractiveness. But it could be instead that they judge him more harshly because they are becoming bored or tired. The attractive condition is always the first condition and the unattractive condition the second.
No comments:
Post a Comment